N7-Methylguanosine (m7G) is important RNA modification at internal and the cap structure of five terminal of message RNA. It is essential for RNA stability of RNA, the efficiency of translation, and various intracellular RNA processing pathways. Given the significance of the m7G modification, numerous studies have been conducted to predict m7G sites. To further elucidate the regulatory mechanisms surrounding m7G, we introduce a novel bioinformatics framework, m7GRegpred, designed to forecast the targets of the m7G methyltransferases METTL1 and WDR4, and m7G readers QKI5, QKI6, and QKI7 for the first time. We integrated different features to build predictors, with AUROC scores of 0.856, 0.857, 0.780, 0.776, and 0.818 for METTL1, WDR4, QKI5, QKI6, and QKI7, respectively. In addition, the effect of window lengths and algorism were systemically evaluated in this work. Our research indicates that the substrates of m7G regulators can be identified and may potentially advance the study of m7G regulators under unique conditions.
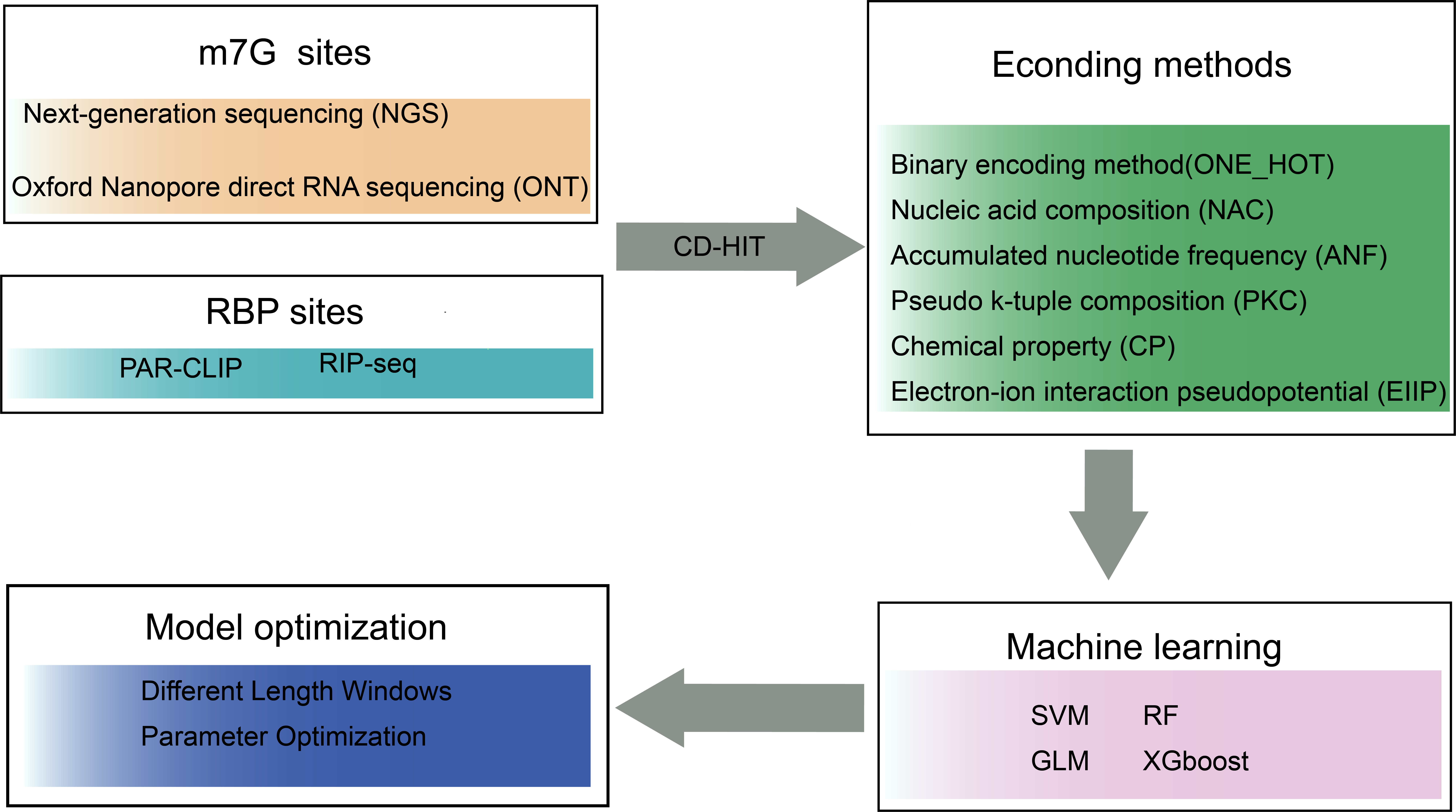
We utilized the 169,718 human m7G sites acquired from m7GHub V2.0 and the binding regions of writers or readers from GEO dataset. Redundant sequences were removed using CD-HIT software with default parameters. Six kinds of encoding methods were considered in the project. The use of SVM is then justified by comparing four machine learning algorithms. Finally, model optimization is performed on the SVM model.
3.1. Features and Performances
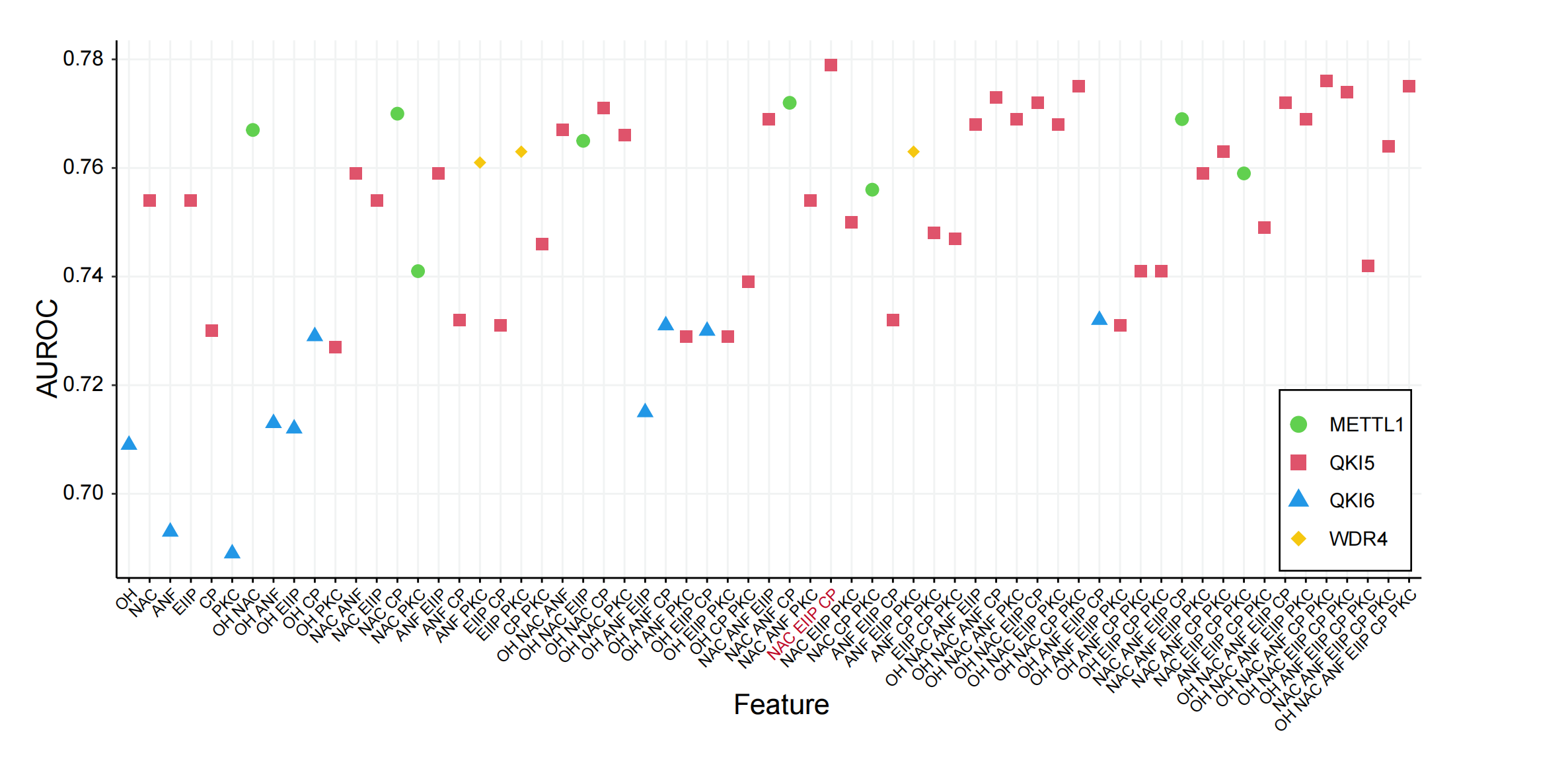
We performed the modeltraining on the training data, followed by validation on the independent test data, to determine the best features predicted by the m7G regulators by calculating the AUROC values. We considered all possible combinations of features across six encoding schemes, ranging from single features to multiple feature combinations. The results indicated that the feature combinations from the CONPOSI, EIIP, and ChemProper encoding methods exhibited the best average performance in the prediction of regulators and substrates. We demonstrate the average performance (AUROC) of various feature combinations in the prediction of substrate regulation across several substrates. Consequently, we selected the CONPOSI, EIIP, and ChemProper encoding methods to construct the preliminary m7GRegpred, The three-feature combination and selection framework demonstrates higher accuracy,surpassing the performance of any Individual sequence-derived feature. The AUROC scores of each model are shown in the table.
3.2. Performance of Different Length Windows
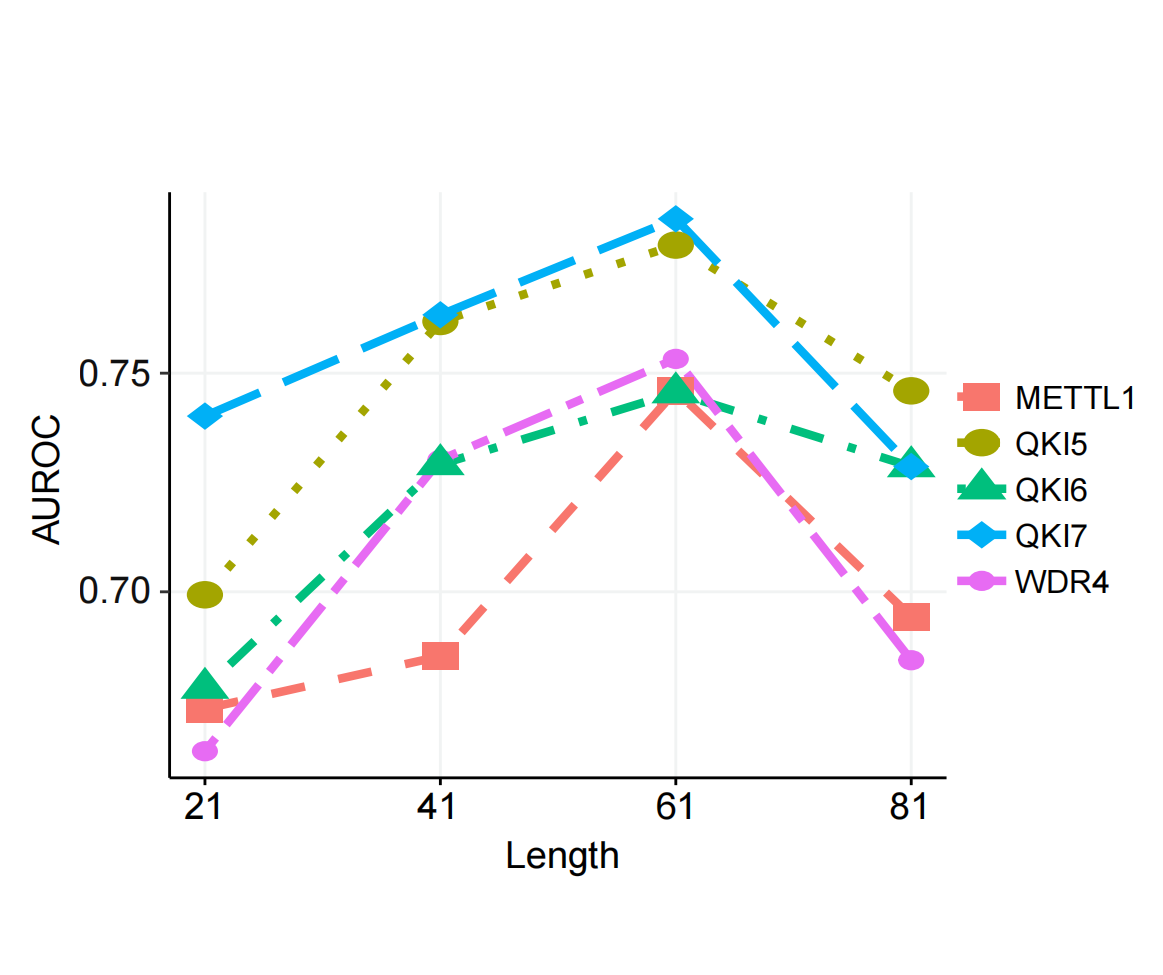
Different lengths of sequence window lengths contain different amounts of sequence information, and the choice of length directly affects the performance of the trained predictor. Therefore, we chose reasonable length of the input sequence after comprehensive consideration. We tested sequences centered on the m7G modification site with lengths of 21, 41, 61, and 81 nucleotides (nt) to determine the optimal predictive results. In the full transcriptome model, the substrate prediction performance for several regulators initially improved.As the length increased, the AUROC score reached its highest value, and then the AUROC score gradually decreased or leveled off. Based on these results, we selected a sequence length of 61 nt in the full transcriptome model for several regulators to generate features.
3.3. Algorisms and Evaluation
Support vector machines (SVMs) have been widely recognised and applied in the field of RNA modification prediction due to their excellent prediction accuracy and generalisation ability. In order to confirmed that SVM is a more suitable machine learning algorithm for our project to perform prediction of substrate of regulators, we conducted a systematically comparison with other prominent algorithms, including RF, GLM, and XGBoost. The performance of the predictors was assessed primarily by calculating AUROC on the independent test data, and also by evaluating metrics such as Accuracy, Sensitivity and Specificity to aid judgement of the performance of the predictors In sum, when employing an optimized sequence length, SVM demonstrated the most consistent and superior performance across the board
3.4. Parameter Optimization of the SVM Model
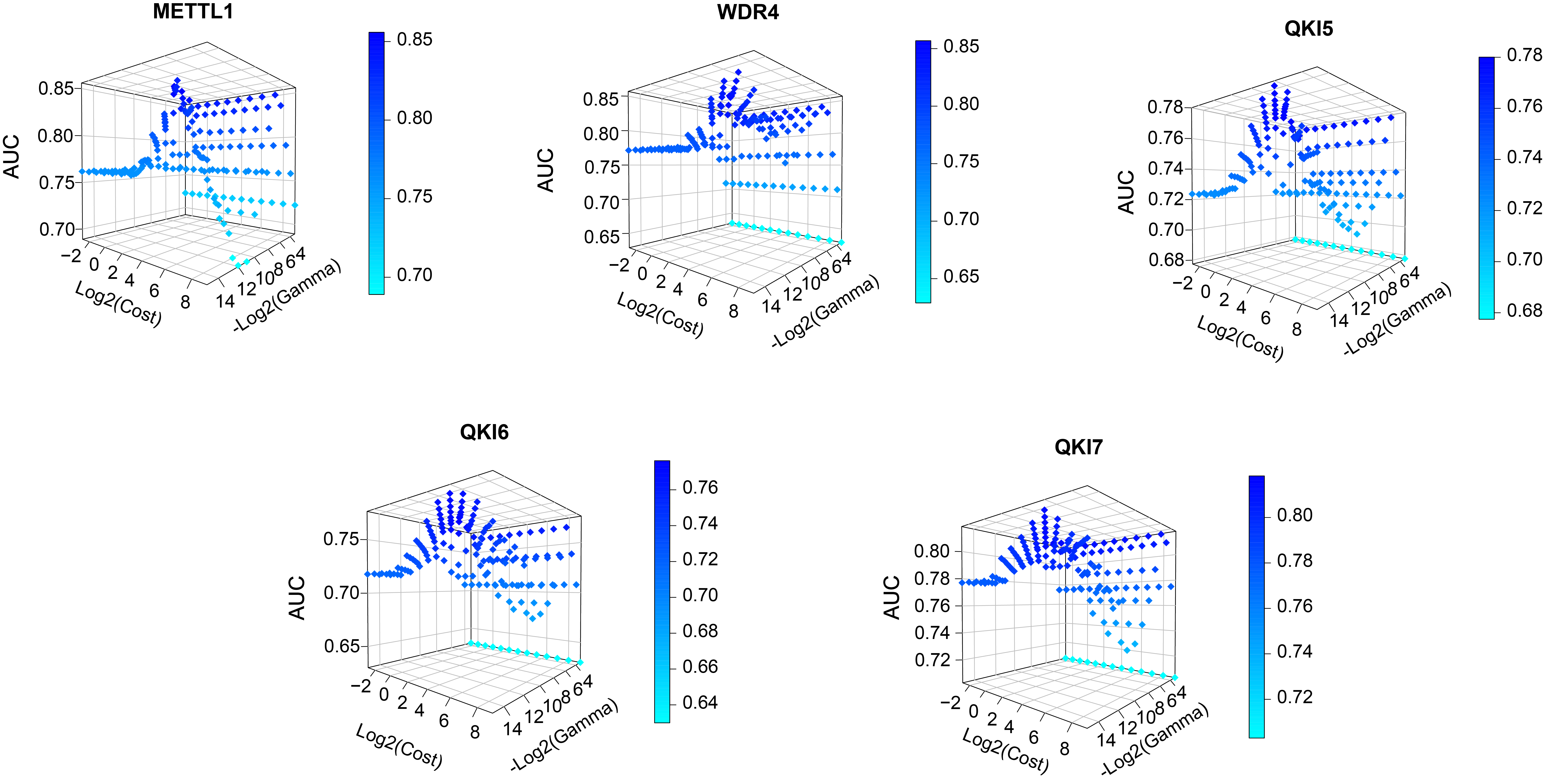
In this study, we combined all the parameter combinations for the C parameter range from 2-3 to 29 and the Gamma parameter range from 2-15 to 2-3 and used this to compare the predictive performance of the models by calculating the AUROC values. Based on the resulting AUROC values, the most appropriate parameter combinations were selected.Specifically, the final optimized model achieved AUROC scores of 0.856, 0.857, 0.780, 0.776, and 0.818 in the independent tests for substrate prediction of the full transcriptome model for METTL1, WDR4, QKI5, QKI6, and QKI7, respectively.
4.1. Input
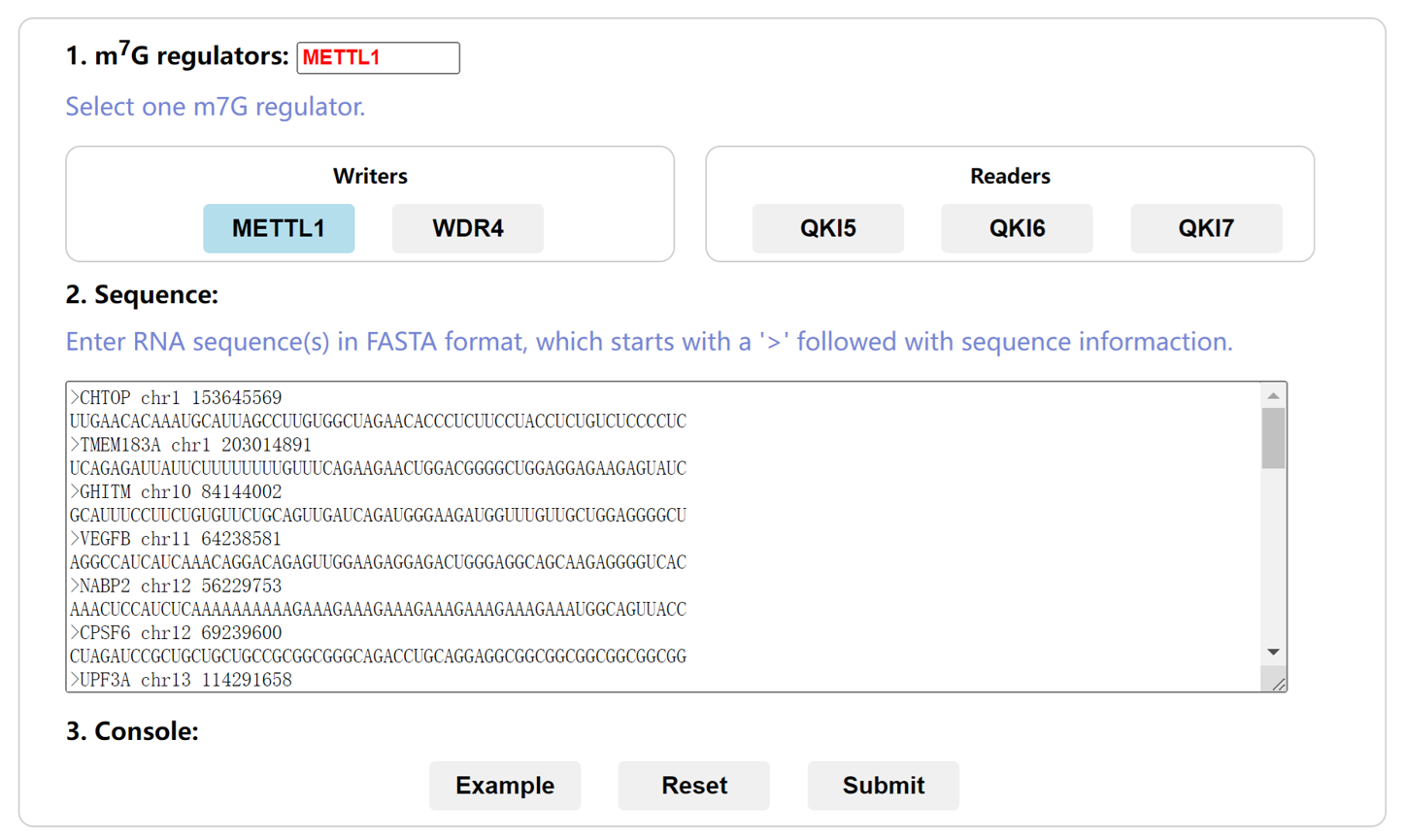
1. m7G regulatos: Users can select different m7G regulator substrate predictors by clicking the button below.
2. Sequence: User can directly copy one or more sites with 61nt length FASTA format in the input box.
3. Console: Users can click on buttons for different functions. Submit, reset the submission form, or access the example dataset.
4.2. Output
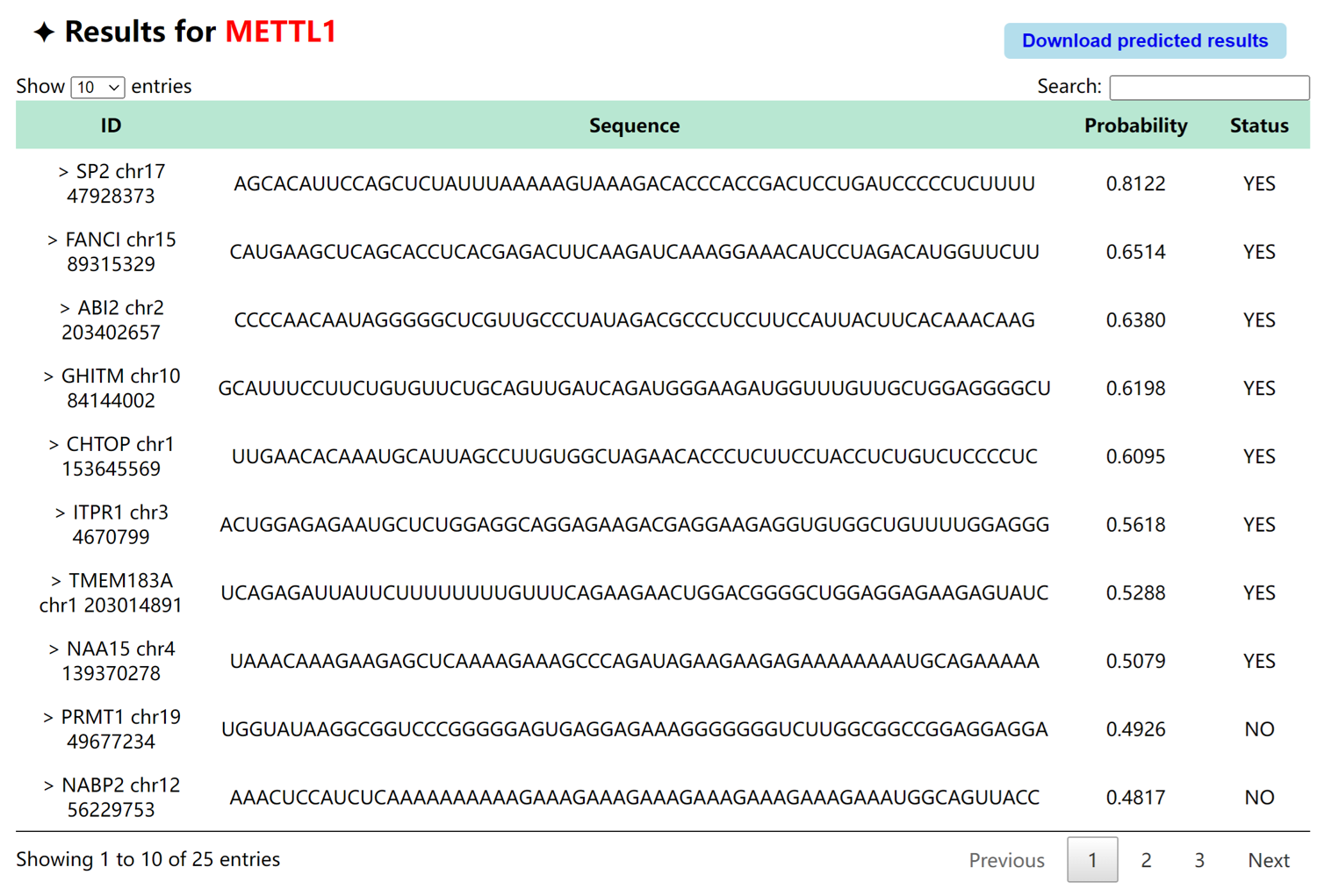
After submitting the data, the prediction results will be visualized with specific information. The information of the prediction consists of two components, "Probability", which indicates the probability of a positive prediction, and "Status", which indicates the actual predicted results. Users can find the specific prediction by searching for the ID, and can also download the prediction results.